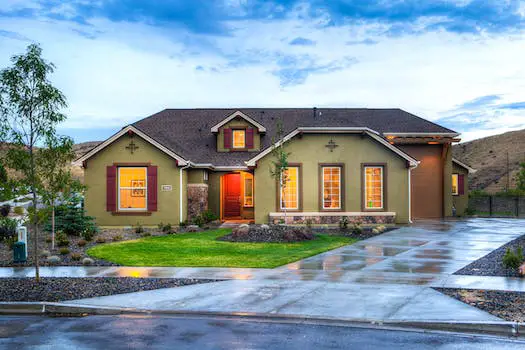
AI-Driven Real Estate Market Forecasting Techniques
The real estate industry is constantly evolving, and with the advent of artificial intelligence (AI), market forecasting techniques have become more accurate and efficient. In this article, we will explore various AI-driven real estate market forecasting techniques that are transforming the industry. We will discuss their benefits, provide examples and case studies, and offer valuable insights for real estate investors, homeowners, first-time home buyers, and real estate agents.
Introduction to AI-Driven Real Estate Market Forecasting
AI-driven real estate market forecasting refers to the use of artificial intelligence and machine learning algorithms to analyze and predict trends in the real estate market. These techniques help professionals make informed decisions about property investments, pricing strategies, and marketing efforts. Some of the most popular AI-driven forecasting techniques include:
Time Series Analysis
Time series analysis is a statistical method used to analyze historical data and identify patterns that can be used to forecast future trends. In real estate, time series analysis can be applied to various data sets, such as property prices, rental rates, and housing inventory levels. By analyzing these data sets, professionals can identify trends and make predictions about future market conditions.
For example, Zillow, a leading real estate marketplace, uses time series analysis to generate its Zestimate home value estimates. By analyzing historical home sales data, Zillow can predict future home values and provide valuable insights to buyers, sellers, and real estate agents.
Neural Networks
Neural networks are a type of machine learning algorithm that mimics the human brain’s structure and function. They consist of interconnected nodes or neurons that process and transmit information. In real estate market forecasting, neural networks can be used to analyze large amounts of data and identify complex patterns that may not be apparent through traditional statistical methods.
A case study by researchers at the University of Southern California demonstrated the effectiveness of neural networks in predicting real estate prices. The study used a neural network model to analyze historical housing data and accurately predicted future price trends, outperforming traditional linear regression models.
Decision Trees
Decision trees are a type of machine learning algorithm that uses a tree-like structure to represent decisions and their possible outcomes. In real estate market forecasting, decision trees can be used to analyze various factors that influence property prices, such as location, property size, and local amenities. By considering these factors, decision trees can help professionals make more informed decisions about property investments and pricing strategies.
For instance, Redfin, a popular real estate brokerage, uses decision trees to predict the likelihood of a home selling within a specific timeframe. This information helps sellers and agents determine the best time to list a property and set competitive prices.
Support Vector Machines
Support vector machines (SVM) are a type of machine learning algorithm that can be used for classification and regression tasks. In real estate market forecasting, SVM can be used to predict property prices and identify trends in the market. SVM works by finding the optimal hyperplane that separates data points into different classes or categories, allowing professionals to make more accurate predictions about future market conditions.
A study published in the Journal of Real Estate Research demonstrated the effectiveness of SVM in predicting residential property prices. The study found that SVM outperformed traditional regression models, providing more accurate and reliable price predictions for real estate professionals.
Random Forests
Random forests are a type of machine learning algorithm that combines multiple decision trees to improve prediction accuracy and prevent overfitting. In real estate market forecasting, random forests can be used to analyze various factors that influence property prices and market trends. By combining the predictions of multiple decision trees, random forests can provide more accurate and reliable forecasts.
A study conducted by researchers at the University of California, Riverside, demonstrated the effectiveness of random forests in predicting real estate prices. The study found that random forests outperformed traditional regression models and provided more accurate price predictions for residential properties.
Conclusion
In conclusion, AI-driven real estate market forecasting techniques are transforming the industry by providing more accurate and efficient predictions. Time series analysis, neural networks, decision trees, support vector machines, and random forests are just a few examples of the innovative techniques being used to analyze and predict market trends. By leveraging these advanced technologies, real estate professionals can make more informed decisions about property investments, pricing strategies, and marketing efforts, ultimately benefiting investors, homeowners, first-time home buyers, and real estate agents alike.
Leave a Reply